Case Study: FlyQuest
1. A HISTORY OF FLYQUEST
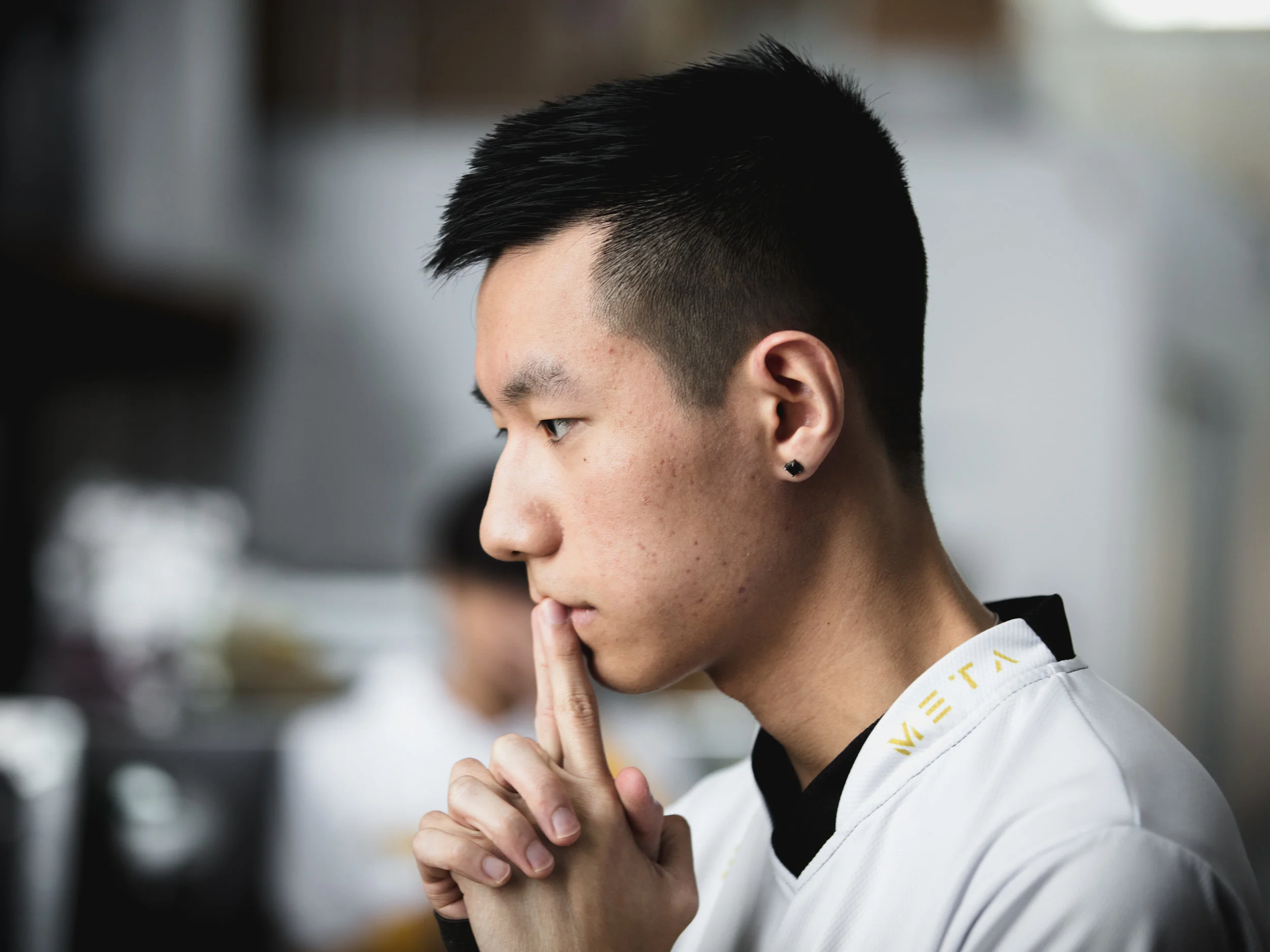
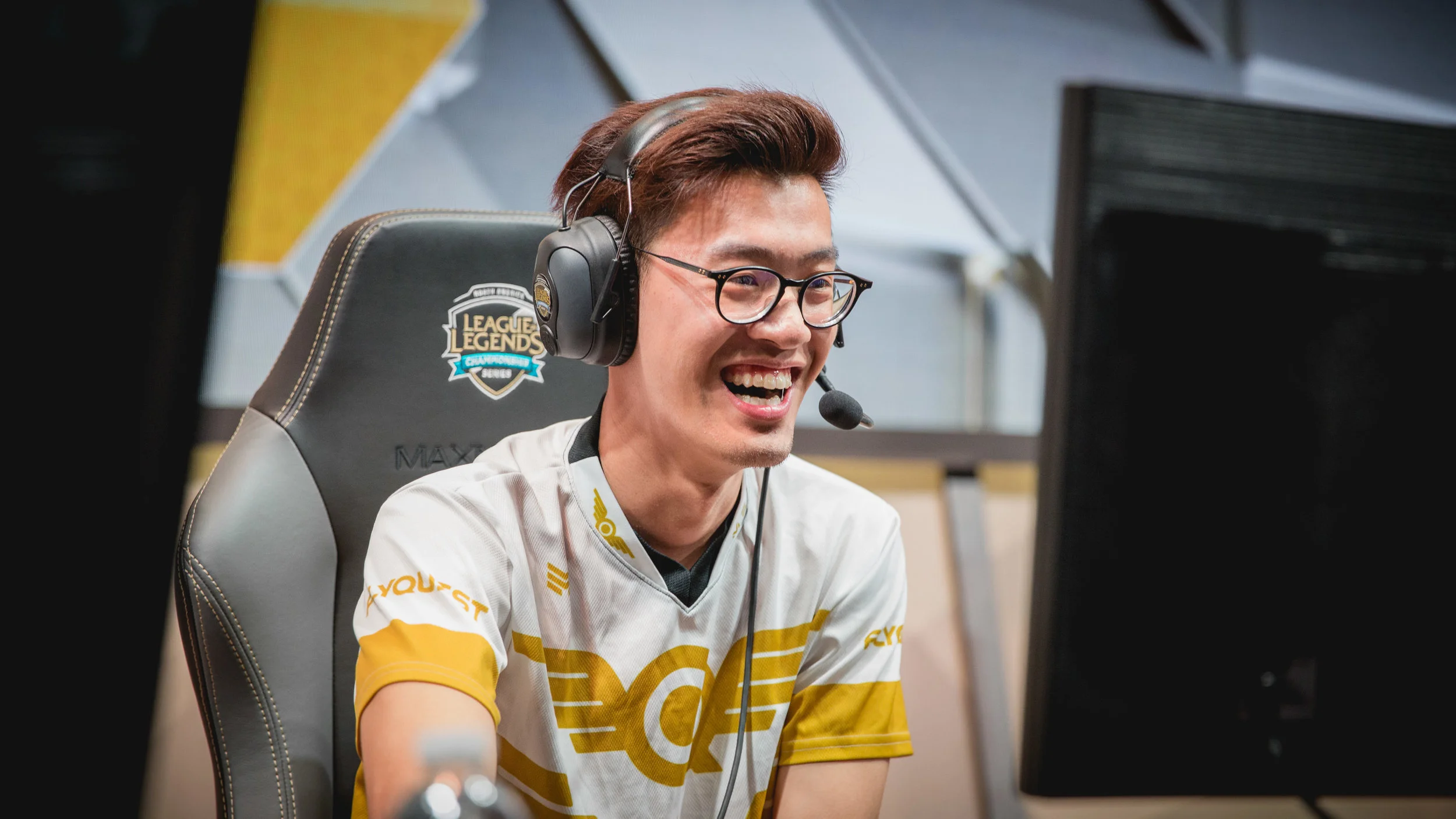
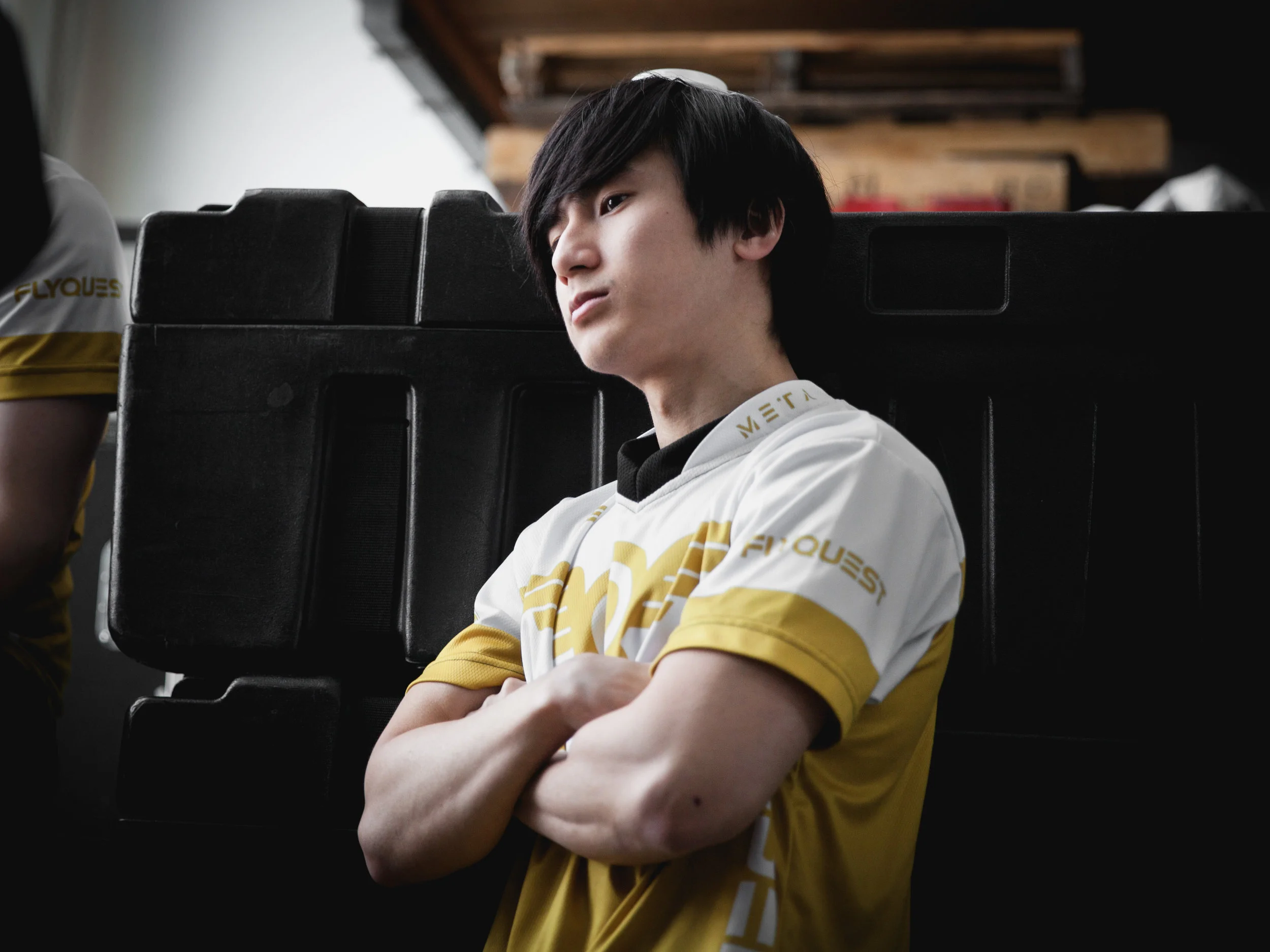


Originally known as Cloud 9 Challenger, FlyQuest entered the North American League Championship Series in the Spring 2017 split sporting LCS Veterans: Balls, Moon, Hai, Altec, and LemonNation. Although they started off strong in the beginning of the split, FlyQuest began to struggle in the second half – losing six out of the remaining nine games. However, they were able to finish off in 4th place after narrowly losing to Phoenix1 in a five game series during the Playoffs.
Following that successful split, Altec left the roster for Team Dignitas, and the vacant position was filled by WildTurtle – formerly of TSM and Immortals. With spirits high and hopes renewed after acquiring a high-profile AD Carry, FlyQuest began the Summer 2017 split. However, things didn’t go as expected, and FlyQuest ended the regular season with a 4:11 W/L record – landing them in 7th place and gaining no championship points for the split.
The team qualified for the Summer 2017 Gauntlet due to their respectable performance in the previous split. With their eyes set on competing at Worlds as North America’s 3rd seed, they started off their gauntlet run against 5th place, Dignitas. In a complete upset, FlyQuest swept the series in a dominating 3:0 fashion and headed into their next game against Counter Logic Gaming. Unfortunately, FlyQuest’s 2017 journey ended in the following round – losing in 4 games to CLG.
2. Goals
We will be attempting to calculate an unbiased numeric value to represent each team members’ contributions to their games as a unit during the Summer 2017 split. Since barons, dragons, and game length also play a contributing factor outside of individual player performance, we will have to include those as factors when creating an accurate model. Once an appropriate model is developed, we can dive further into how FlyQuest functions as a team, where they prosper, as well as where they lack.
3. Methodology
- We will be gathering player statistics based on individual game results, rather than by the winner of the best of three. We can therefore look at each game independently and increase our sample size, as well.
- In an effort to create both a uniform and unbiased way of representing each player, we will normalize player statistics based on the entire set of North American LCS players by role. Once normalized, an index score will be created based on selected performance metrics that are not limited by role and will not result in collinearity (i.e. using percent gold share vs total gold). This method will also be applied to dragons, barons, and game length.
- Opponent strength also has a factor in this analysis. The teams will be ranked and scored based on their win percentage and FlyQuest’s win percentage as a benchmark. This value will be applied as a weight to player index scores. Naturally, the first-place team will have the highest score (X>1) while the last-place team will have the lowest score (X<1).
- Wins and losses will be represented binomially with 1 being assigned to wins, and 0 being assigned to losses.
- A logistic regression will be performed by using the scores developed above and the results of the game to develop a model that will predict the likelihood that a game was won or lost. This model will be compared to the actual results of said games.
- Once an acceptable model is created – we will finally be able to determine the strength of association between individual player performance, dragons, barons, and game length to the match results.
4. Caveats
- There are five roles within the game and it’ll be difficult to accurately represent them in a unbiased and uniform way as each role has different defining traits depending on player style and team composition.
- Due to the complexity of League of Legends, there are a multitude of explanatory variables that go into determining how well a player performs – and there are even more that contribute to the match result. With more variables, we increase the likelihood that we introduce collinearity, which would cause unwanted over-adjustment to our model. We attempted to limit the amount of variable used in this model by creating a single index score for each player. Variables would have to be thoroughly checked before being introduced to the regression.
- Numbers don't tell the entire story - as much as we tried to incorporate variables that were deemed influential, play-styles, jungle proximity, and other categorical descriptors can not be represented numerically.
5-1. Model Results
- Our model was able to confidently predict 18 out of 21 wins, and 29 out of 31 losses.
- Out of the remaining 3 games in the set of wins, the games against Team EnvyUs (Week 4, Game 1) and Echo Fox (Week 5, Game 2) were strongly predicted as losses, while the game against Team Liquid (Week 8, Game 1) could have swung either way.
- Out of the remaining 2 games in the set of losses, the game against Echo Fox (Week 5, Game 1) was strongly predicted as a win, while the game against CLG (Week 3, Game 3) could have swung either way.
Figure 1.1
From our regression coefficients in Figure 1.1, we can conclude that Hai has the highest influencing coefficient out of the other players. As someone renowned for his shot calling and team leadership – it shouldn’t be surprising that he holds the strongest influence towards the result of the game. Following in a close second, is WildTurtle in the AD Carry role, with LemonNation, Moon, and Balls far behind. Based on the coefficients, it seems that FlyQuest either likes to play towards their carries or is too reliant on them to win matches.
Figure 1.2
According to Figure 1.2, this team utilizes Dragon almost twice as effectively as Baron, and has a slight disadvantage as game time presses on – however, at a -0.062 coefficient, this isn’t a major contributing factor for game results.
5-2. overall game Performance
Figure 2.1
Number of Games: 21
Average Game Time: 36.91 min
Figure 2.2
Number of Games: 30
Average Game Time: 34.74 min
By splitting the games so we can look at the wins and losses independently, we can see that based on Figures 2.1 and 2.2, WildTurtle is the best performing member during wins, while Moon takes that mantle during losses. However, since all members’ performance scores are well within range of each other it can be deduced that not one person is significantly better than the other – FlyQuest is a well balanced team.
5-3. game performance by Wins and losses
Diving further into FlyQuest’s wins and losses by graphing out their performance scores per game by result, we can backup our resulting influencing coefficients by visualizing how important Hai and WildTurtle are to the success of the team.
Figure 3.1
We observe in Figure 3.1 that the team performed quite well during their wins (for the most part). However, more can be learned from a team's failures rather than successes.
Figure: 3.2
And Figure 3.2 does that for us by telling another side of the story that helps emphasize the importance of the two carries. Aside from the outlier against Echo Fox, even with Balls, LemonNation, and Moon playing above average in many of the games, they can barely influence the result of the match if Hai and WildTurtle don’t do the same. In fact, Hai and WildTurtle have under-performed in the majority of the losses.
These two graphs also add another spotlight on the team, as it shows that Moon is both a reliable and consistent player for FlyQuest – holding the best average performance score during their losses, on top of keeping up with the four senior veterans during their wins. However, due to the lack of converting Moon’s performance to wins, our regression netted him the 4th lowest influencing coefficient of the 5 players.
5-4. game performance by Opponent
Figure 4
Of all their losses, FlyQuest has only dropped four games to the lower tier teams (3 to Echo Fox, 1 to Team Liquid), and can trade blow for blow with the middle of the pack teams. Unfortunately, this team falls short when it comes to their head-to-head against the top four teams of the league, only being able to take a total of four games (2 off Immortals, 2 off CLG). However, it should be noted that FlyQuest actually had a losing record to Team Dignitas throughout the regular season; they were only able to obtain a winning record after a 3-0 upset against them in the regional qualifiers.
5-5. game performance during regional qualifiers
Ending the analysis off, we look at their regional qualifier performance, which many fans have praised FlyQuest – especially WildTurtle – for noticeable improvement in their play from the regular season, even during their loss against CLG.
Figure 5
We can observe that Figure 5 provides further reinforcement that WildTurtle was playing at a higher level by performing near and above expectations in 6 out of the 7 playoff games.
However, one player whose performance has been overlooked during the regional qualifiers is Moon’s. Similar to WildTurtle, Moon has also performed near and above expectations in 6 out of the 7 games.
Despite the improved performances from both FlyQuest’s AD Carry and Jungler, a glaring weakness of FlyQuest is revealed in that they are still heavily reliant on both of their two carries to perform well in order to net a win. It would seem that they have not differentiated from that style during the 1 month LCS hiatus between the regular season and the regional qualifiers. While Figure 5 shows that almost everyone under-performed during the 4 game series against CLG, we can't forget that Hai has the highest influencing coefficient out of all his teammates. Despite their best efforts, Hai’s under-performance during all 4 games hindered FlyQuest from advancing to the final round; even with early leads in Games 3 and 4, he couldn't translate his advantage to a win.
6. conclusion
FlyQuest's road to a 5th place finish was a long and hard fought journey. However, despite their rocky start and end to the regular season, FlyQuest was able to capitalize on the 1 month break they had before regional qualifiers began in order to improve on their strengths and play towards Hai and WildTurtle. While it was probably too late to learn to play towards different parts of the map, the analysis shows that they have a potential carry in Moon. Looking back to FlyQuest's earlier games during the Spring 2017 split, we are reminded that the phrases "It's Hai Moon" and "Fly Hai to the Moon" were coined because of Moon's emphasis in getting Hai and – subsequently – himself ahead. Emphasizing mid lane priority and jungle control could be a potential route for them to experiment in again since, this time around, the dynamic would be different with WildTurtle rather than Altec in the AD Carry position. It's fairly evident that FlyQuest has improved significantly and that they can perform even better by playing towards their mid laner and AD Carry, but given the small sample size of games after the hiatus, we can't be certain that they will sustain this trend. Nevertheless, this 5 man squad has a bright future, and I look forward to cheering them on in 2018.
7. Legality
Disclaimer: I am not endorsed by Riot Games nor FlyQuest, and do not reflect the views or opinions of Riot Games, FlyQuest, or anyone officially involved in producing or managing League of Legends and the FlyQuest Team. League of Legends and Riot Games are trademarks or registered trademarks of Riot Games, Inc. League of Legends © Riot Games, Inc. FlyQuest is a registered trademark of Capture the League LLC.
8. Credits
Photo Source: LoL E-Sports' Flickr, League Of Legends, FlyQuest
Data: Oracle's Elixer